When good data goes bad: Recognizing and preventing data poisoning
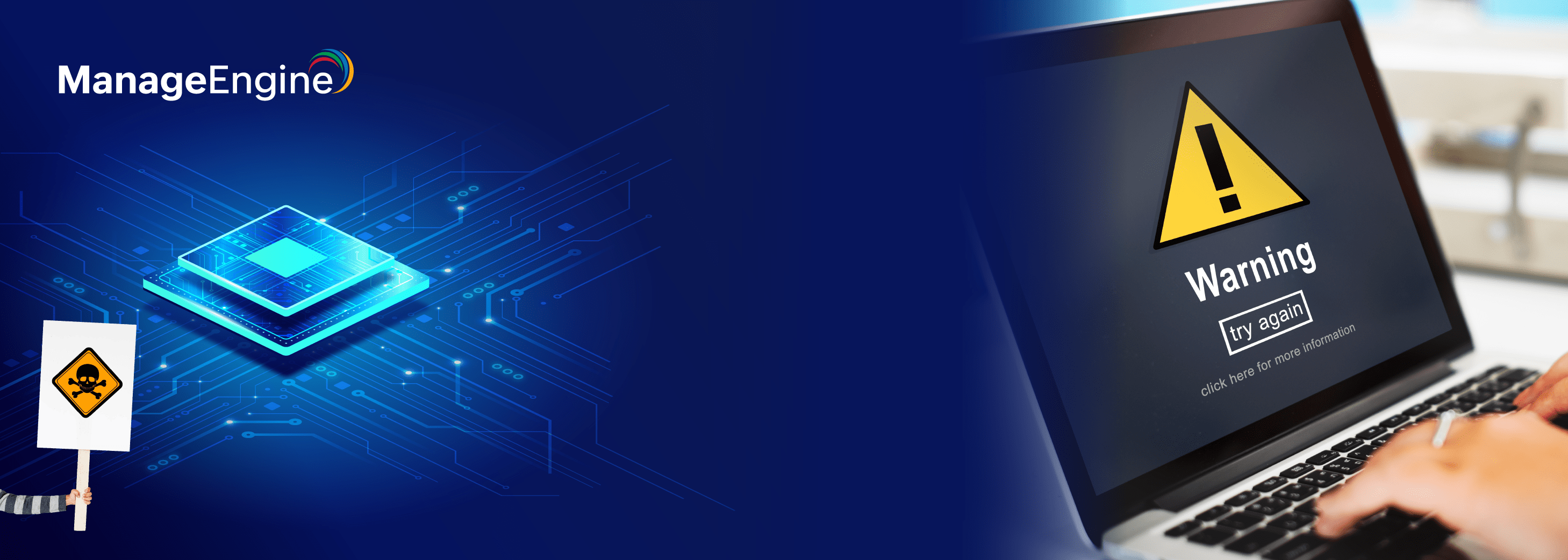
Data integrity is critical in an era where data drives innovation and decision-making. The proliferation of data poisoning, a sneaky and frequently disregarded cyberthreat, puts information trustworthiness in serious danger.
Unseen threats in the digital realm
Data poisoning involves the manipulation or contamination of datasets, introducing malicious elements that compromise the accuracy and effectiveness of algorithms, machine learning models, and decision-making processes. This covert attack method exploits the trust placed in data systems, leading to skewed outcomes, flawed predictions, and potentially catastrophic consequences.
The mechanics of data poisoning: A stealthy intruder
Fundamentally, data poisoning is the introduction of false or deceptive information into authentic datasets to compromise systems. Attackers exploit vulnerabilities in data collecting procedures, frequently taking advantage of shoddy security protocols, unprotected endpoints, or compromised user inputs. The goal is to contaminate the data that algorithms use to learn, causing them to draw erroneous conclusions and judgements.
Recognizing the signs of data poisoning: Red flags to watch for
1.Outliers and anomalies: Unusual patterns or extreme values within datasets may indicate manipulated or poisoned data.
2. Inconsistencies in predictions: A sudden decline in the accuracy of machine learning models or unexpected results can signal the presence of poisoned data.
3. Unexplained model biases: If a model displays biases that cannot be attributed to natural variations, it may be under the influence of poisoned data.
4.Unexpected behavior in real-world applications: Discrepancies between predicted outcomes and actual results in real-world scenarios may suggest data poisoning.
5.Abnormal user inputs: Anomalies in user-generated data, especially in systems heavily reliant on user inputs, can be a red flag for data poisoning.
The implications of ignoring data poisoning: Risks and consequences
Failing to recognize and address data poisoning can have severe consequences. Inaccurate forecasts and choices can result in monetary losses, jeopardized security, and reputational harm to a company. The hazards are significantly greater in crucial industries like healthcare, banking, and autonomous systems, with possibly fatal consequences.
Strategies for recognition and prevention
1.Implement robust data validation: Regularly validate incoming data to detect anomalies and ensure its integrity before it influences algorithms or models.
2.Adopt anomaly detection techniques: Employ anomaly detection algorithms to identify unusual patterns and outliers within datasets.
3.Perform continuous monitoring and model evaluation: Regularly monitor and evaluate machine learning models for unexpected biases, inaccuracies, or shifts in performance.
4. Diversify data sources: Rely on a diverse range of data sources to reduce the risk of poisoning attacks that target specific datasets.
5. Establish user education and awareness: Educate users and data contributors about the potential risks of providing inaccurate or manipulated data.
6. Implement strong access controls: Restrict access to critical data repositories, and implement strong access controls to prevent unauthorized manipulation.
7.Regularly update security measures: Stay vigilant and update security measures to address new vulnerabilities and evolving threats in the data landscape.
The reliability of data-driven systems is clearly at risk from data poisoning. To protect the integrity of digital insights, it is critical to identify the warning symptoms of data poisoning and take preventative action to lessen its effects. In a time when data is transforming how we perceive the world, it is the duty of individuals, institutions, and the cybersecurity community as a whole to safeguard it. By staying informed and adopting robust security practices, we can collectively ensure that good data remains a trustworthy foundation for innovation and decision-making in the digital age.