Five worthy reads is a regular column on five noteworthy items we’ve discovered while researching trending and timeless topics. This week, we explore the concept of XAI, Explainable AI.
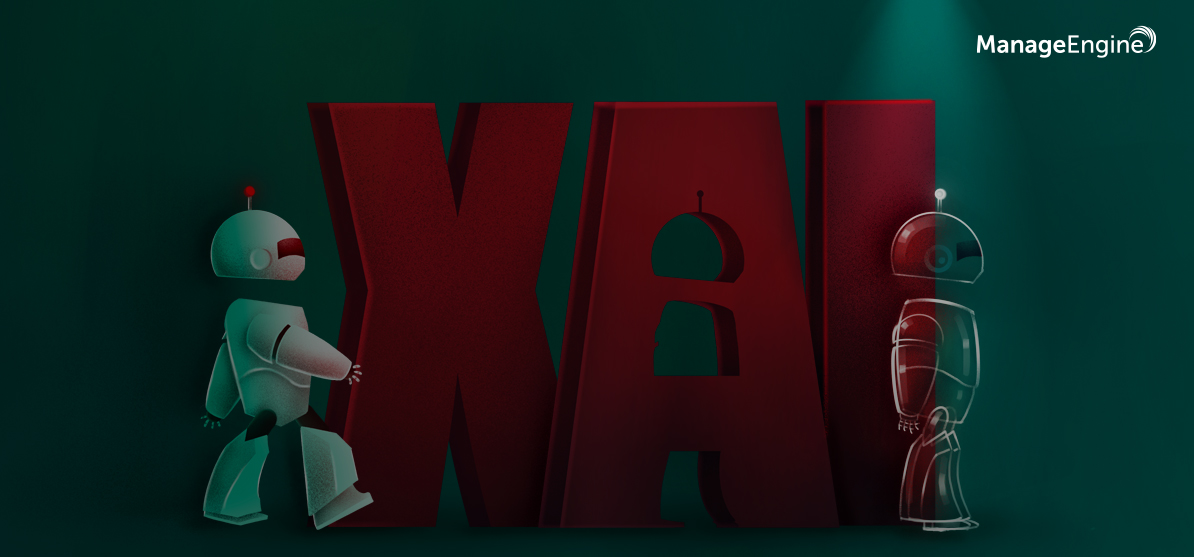
Illustration: Dorathe Victor
Have you ever wondered about the intricate logic behind sponsored content and recommendation systems found across social media and online retailer pages? With the significant advances in artificial intelligence (AI) technologies, we now interact with AI-enabled systems on a daily basis, on both personal and professional levels. From the image and voice recognition systems on our mobile phones to the complex decision-making systems used by researchers, policy-makers, medical professionals, bankers, etc., the scale of AI use is increasing with no end in sight.
AI tools today are capable of producing highly accurate results, but are also highly complex. They use machine learning (ML) models that are opaque and non-intuitive, aptly called black-box models. Users have little to no knowledge about the process behind the decision-making and predictions provided by these tools. When data is fed to the AI tool, there is no explanation offered for the results it produces; this lack of explainability obstructs peoples’ ability to trust AI, eventually leading to distrust in the decisions provided by these tools.
White-box AI, or Explainable AI (XAI), addresses this issue by offering a level of transparency to users and providing explanations about the reasoning behind decisions. This model helps users understand why an AI system works a certain way, assesses its vulnerabilities, verifies the output, and safeguards against unintentional biases in the output. XAI also makes adhering to regulatory standards or policy requirements a piece of cake; data regulations like the General Data Protection Regulation (GDPR) and the California Consumer Privacy Act (CCPA) grant consumers the right to ask for an explanation about an automated dispute, and XAI can easily provide this data.
“Gartner predicts that ‘by 2023, 40 percent of infrastructure and operations teams will use AI-augmented automation in enterprises, resulting in higher IT productivity. As companies proceed from narrow AI to general AI—and begin automating not only processes but also decisions—it’s vital that AI tools explain their behavior,'” says Ramprakash Ramamoorthy, product manager at Zoho Labs.
Now that we know what XAI means and does, here are five interesting reads that further discuss XAI.
1. Understanding Explainable AI
Different AI models require different levels of explainability and transparency. These levels should be determined by the consequences that can arise from the AI system, and businesses should implement governance to oversee and regulate the system’s operations.
2. How Explainable AI Is Helping Algorithms Avoid Bias
Sometimes, subtle and deep biases can creep into the data fed into complex AI algorithms. Making AI models increasingly more explainable is key to correcting the factors that inadvertently lead to bias.
3. Explainable AI in healthcare
AI explainability is particularly critical in the healthcare industry. The sorts of decisions and predictions made by AI-enabled systems here are critical to life, death, and personal wellness. XAI reduces uncertainties in the outcomes, making things less risky.
4. Why explainable AI is a critical business strategy
For businesses, XAI aids in better governance. When the decision-making process becomes transparent, being compliant with regulations is easier and less problematic. This also paves the way towards aligning AI-based decisions with a company’s ethical and business goals.
5. Why asking an AI to explain itself can make things worse
Every coin has two sides. Like every other technology, researchers say, XAI also has its shortcomings. Figuring out the best ways for the AI to explain itself can help prevent users from overtrusting AI.
To put it simply, before you get into a self-driving car, you want to know how it works to ensure it’s safe. In the event that a self-driving car gets into an accident, a law official needs to know what happened in the AI system to cause the accident, and XAI can offer that explanation. The knowledge behind why the car failed to avoid an accident is a valuable lesson to learn for both humans and AI.